<Your Business Headline>
Business Overview
<Your business description>
Geographic Area
<Your service area>
Line of Business
<The type of business you are in>
Brands We Carry
<Your list of brands>
Products and Services
<Products and/or services you provide>
Discounts Offered
<Coupons and other discount information you offer>
Additional Information
<Any other information about your business>
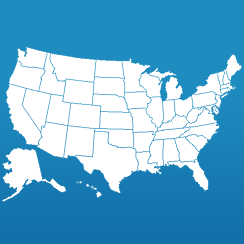
Business Hours
<Your hours of operation>
Timezone
<Your timezone>
We Accept
<Methods of payment you accept>
If this is your Web site, you can customize your business profile from your account at Network Solutions®.
Click here to edit your business profile